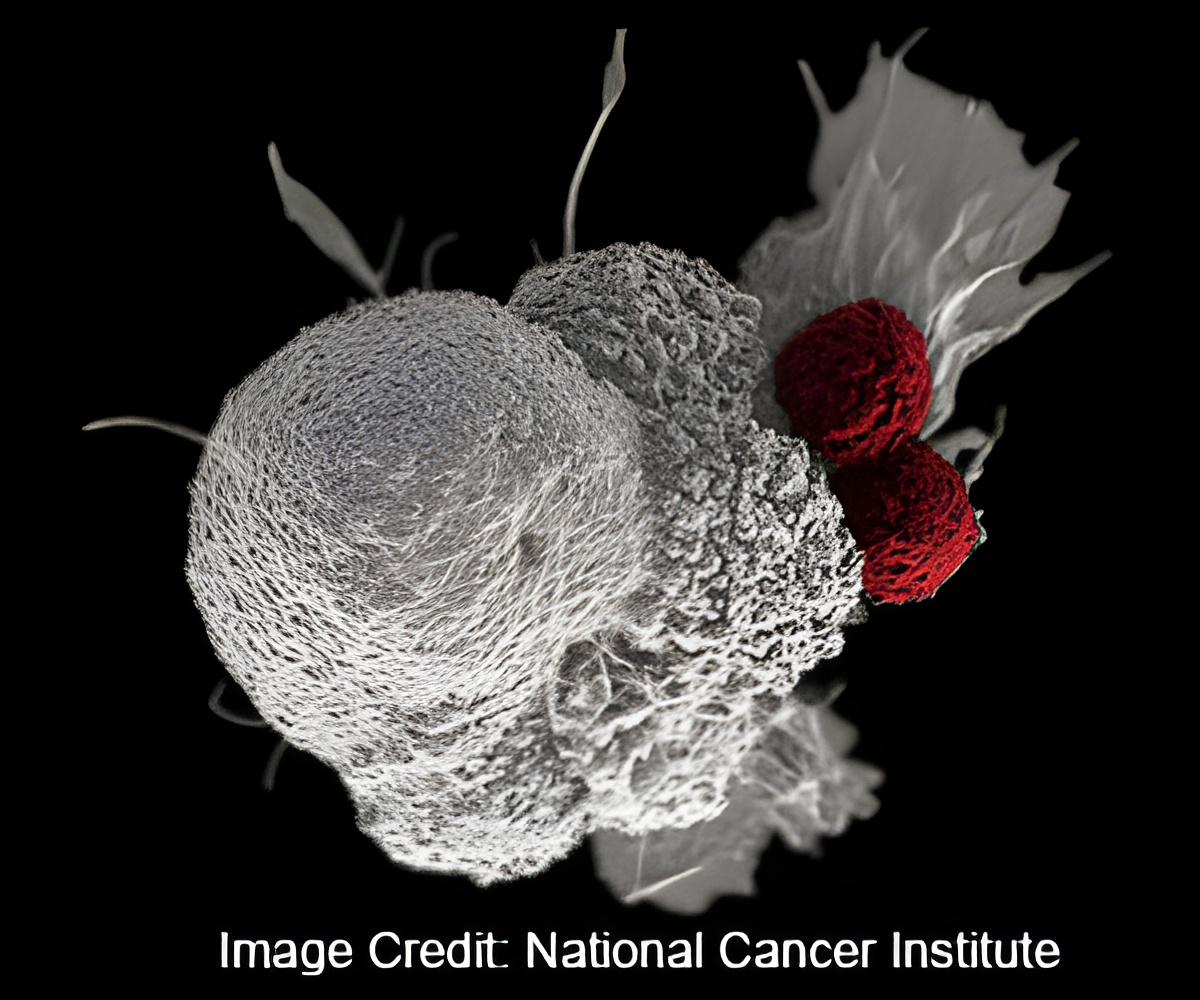
‘A unique feature of the model allows to predict the number of carcinogenesis stages for a subtype of cancer and it also helps to identify the driver mutation associated with different subtypes of cancer.’
Tweet it Now
The progression from one carcinogenesis stage to another is defined by "driver" mutations in crucial genes, which cause the cell to acquire oncogenic properties such as the capacity for accelerated division and apoptosis resistance. Medical statistics show that oncological morbidity and mortality increase with age because carcinogenesis is driven by the accumulation of several consecutive mutations in the DNA.
At the same time, the incidence rates for at least some subtypes of cancer can not only increase but also decrease with age, and this fact cannot be explained in terms of classical oncology.
Many complicated carcinogenesis models involving a variety of assumptions and parameters have been proposed, but none of them provides a way to accurately predict the number of key mutations in a given type of cancer.
So how can a geneticist determine whether a patient is close to accumulating a critical quantity of mutations and if there is a high risk for them to develop cancer?
Advertisement
"A unique feature of the model is that it considers the random nature of mutations and allows to predict the number of key mutations required for the carcinogenesis process," says Belikov.
Advertisement
In the future, it may find utility in clinical practice as well. For instance, a geneticist will be able to find out a patient's cancer risk by studying the number of driver mutations identified by DNA analysis.
Source-Eurekalert