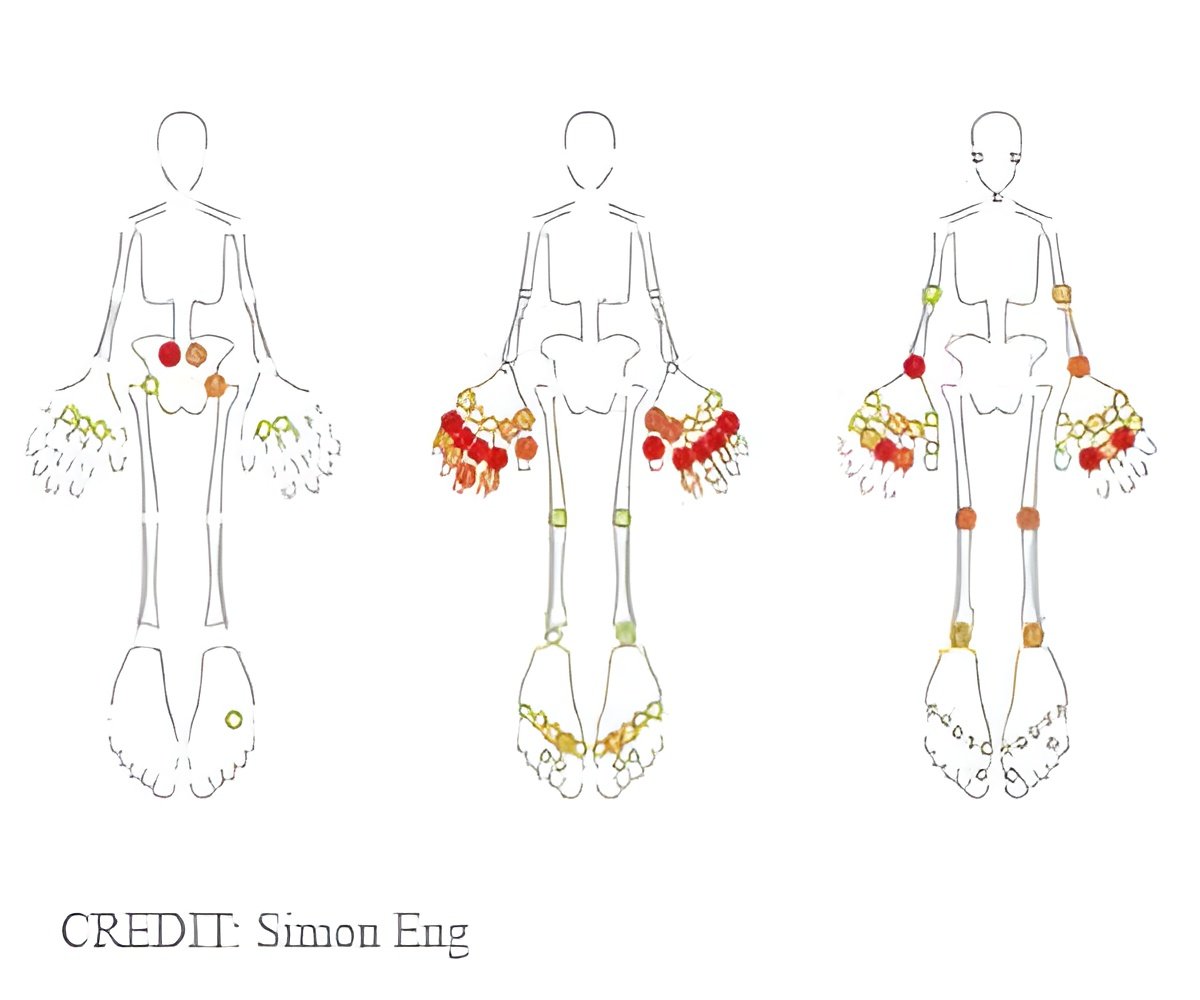
‘Newly developed machine learning tool could help health professionals to spare unnecessary treatments for children with arthritis.’
Read More..Tweet it Now
That could now change thanks to a machine learning tool developed by Quaid Morris, a professor of computer science at the Donnelly Centre for Cellular and Biomolecular Research at the University of Toronto, Dr. Rae Yeung, Professor of Paediatrics, Immunology and Medical Science at the University of Toronto, and their recently-graduated, co-supervised student Simon Eng.Read More..
Morris is also faculty in the Vector Institute for Artificial Intelligence and is an inaugural AI Chair by the Canadian Institute for Advancement of Research. Yeung is also the inaugural Hak-Ming and Deborah Chiu Chair in Paediatric Translational Research at the Hospital for Sick Children (SickKids).
The research team describes a computational approach based on machine learning, a form of artificial intelligence (AI) in which the computer learns to recognize recurrent patterns from a set of data. The algorithm was able to classify patients into seven distinct groups according to the patterns of swollen or painful joints in the body. Moreover, it also accurately predicted which children will go into remission faster and which ones will develop a more severe form of disease.
An estimated 300,000 children are suffering from arthritis in the US alone. While its triggers still remain unclear, the disease occurs when the immune system mistakes the body's own cells for foreign invaders, attacking the lining of the joints to cause swelling, pain and possibly long-lasting damage. There is no cure and the treatment consists of progressively more aggressive and costly medications, starting with anti-inflammatory pain relief drugs, such as ibuprofen, to stronger drugs including methotrexate (a chemotherapy agent), steroids, and biological agents (such as anti-TNF and anti-IL-1) that switch off parts of the immune system.
"The final stage of treatment is very effective in some children, but also very expensive, and it's not clear what the long-term effects are," says Morris. "When you are inhibiting the function of the immune system, this type of treatment can be associated with potential side-effects including increased risk of infection and others"
Advertisement
As a first step, the researchers set out to subtype the children who developed arthritis but had not been treated with medications yet. They analyzed clinical data from 640 children, collected between 2005 and 2010 as part of the pan-Canadian study Research in Arthritis in Canadian Children, Emphasizing Outcomes (ReACCh-OUT). All children received detailed physical examinations as part of their care which included documenting the location of painful (also known as active) joints in the body.
Advertisement
Although unique patterns of joint involvement are recognized at the bedside, the current patient classification for childhood arthritis only takes into account the overall number of affected joints. It is clear that better descriptions of joint involvement are needed that predict disease course and disease severity. It was striking from the data that children with non-localized joint involvement are different. Physicians had already observed this before as they were treating these children with strong medications but were still not able to control the disease.
"Identifying this group of children early will help us target the right treatments early and prevent unnecessary pain and disability from ongoing active disease," says Yeung.
Because of the complexity of the disease, with multiple joints affected and in a way that can change over time, as well as a relatively small number of patients available, the team had to look beyond standard statistical methods to detect patterns of joint pain.
"We had to use machine learning just to detect these seven patterns of disease in the first place," says Morris, whose team modified the technique known as multilayer non-negative matrix factorization.
"And then we realized there are some children who do not fall into any of the patterns and they have a very bad version of the disease. Now we understand the disease much better we can group children into these different categories to predict response to treatment, how fast do they go into remission and whether or not we can tell they are in remission and remove therapy."
Source-Eurekalert