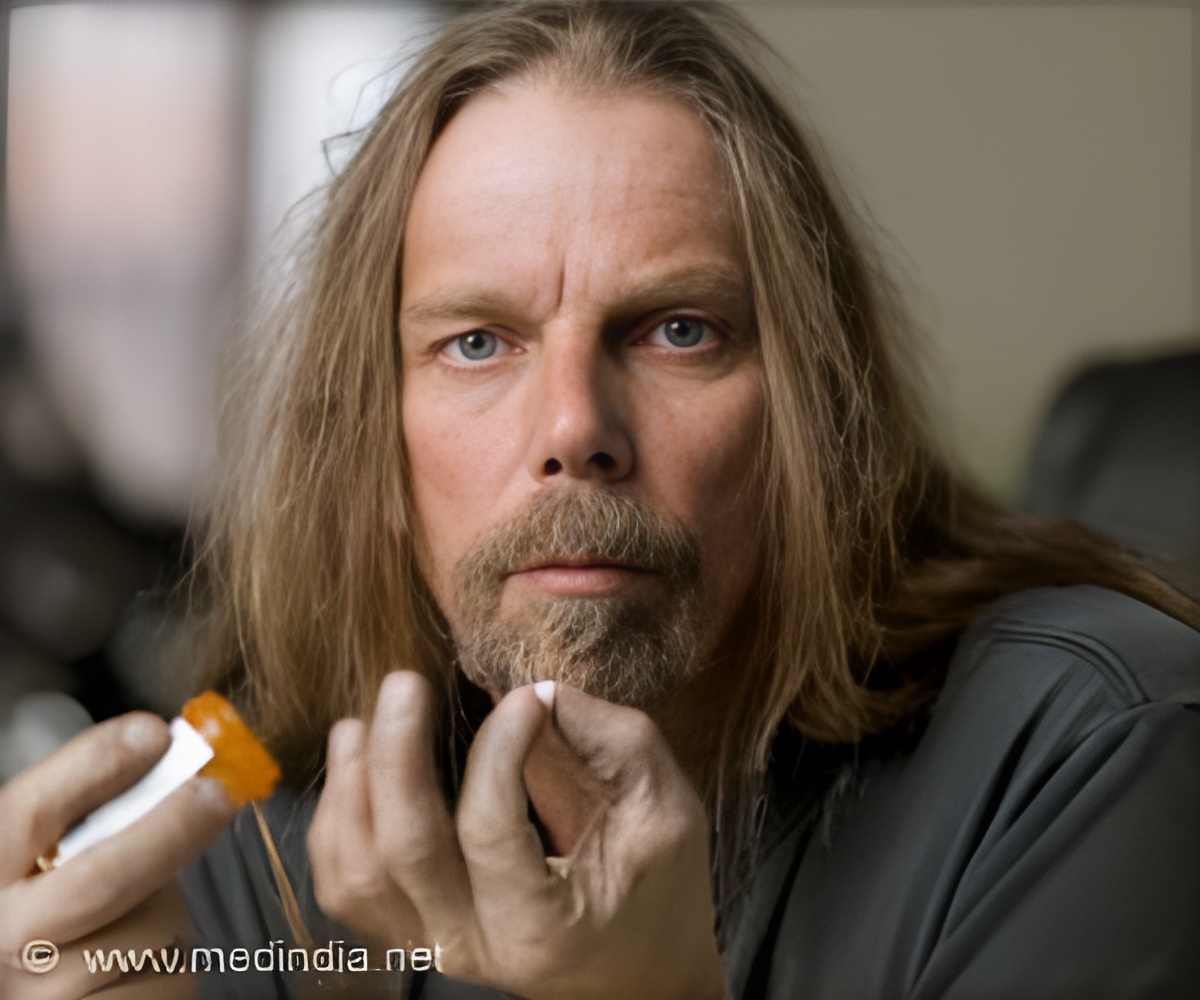
‘Selectively identifying patients who typically require high doses of opioids after surgery is important to help decrease opioid misuse.’
Tweet it Now
Currently, physicians are using questionnaires to identify patients at higher risk for severe post-surgical pain, which is time-consuming. Hence, a more effective method of using machine learning is sought by researchers in this study. They created 3 machine learning models that analyzed patients' electronic medical records, which identified that younger age, female gender, pre-existing pain, higher body mass index, and prior opioid use were the most predictive factors of post-surgical pain."We plan to combine the models with our electronic medical records to provide a prediction of post-surgical pain for each patient," reports Mieke A. Soens, the lead author of the study. "If the patient is identified to be at high risk for severe post-surgical pain, the physician anesthesiologist can then modify the patient's anesthesia plan to maximize non-opioid pain management strategies that would decrease the need for opioids after surgery."
In the two-part study, the research team looked at data from 5,944 patients who had a wide range of surgeries, including gallbladder removal, hip replacement, prostate surgery, and hysterectomy. Of those, 22 percent had consumed 90 morphine milligram equivalent (MME) in the first 24 hours after surgery, which is a high dose.
They used 163 possible factors to predict high pain post-surgery, based on a consultation with experts and literature search. From there, they created 3 machine learning algorithm models (logistical regression, random forest and artificial neural networks) that searched the patients' medical records and shaped the 163 predictor factors down to those who most precisely predicted patients' pain severity and potential opioid needs after surgery.
In the second part of the study, they compared what the models predicted to actual opioid use in those same patients. They determined all 3 models had similar predictive accuracy overall: 81 percent for logistical regression and random forest methods and 80 percent for artificial neural networks. That means the models precisely identified which people were more likely to have severe pain and need higher doses of opioids about 80 percent of the time.
Advertisement
Source-Medindia